Generative AI is reshaping our digital landscape, enabling machines to create new content from art to code. This guide cuts through the complexities to offer insight into the mechanisms and impact of generative AI, its diverse applications, and the critical challenges it poses for society. Get equipped to understand the transformative power of generative AI, setting the stage for future advancements.
Key Takeaways
- Generative AI can produce diverse content, such as text, images, and audio, using advanced Transformers and Diffusion models and holds transformative potential across various domains, including content creation, software development, and healthcare.
- While generative AI presents wide-ranging applications and benefits, it poses significant challenges, such as biased outputs, security risks, and substantial energy consumption, raising sustainability concerns.
- To effectively utilize generative AI, following best practices that ensure transparency, maintain human oversight, protect data privacy, and address proprietary concerns is crucial. These measures build trust and promote responsible usage.
Exploring the Foundations of Generative AI
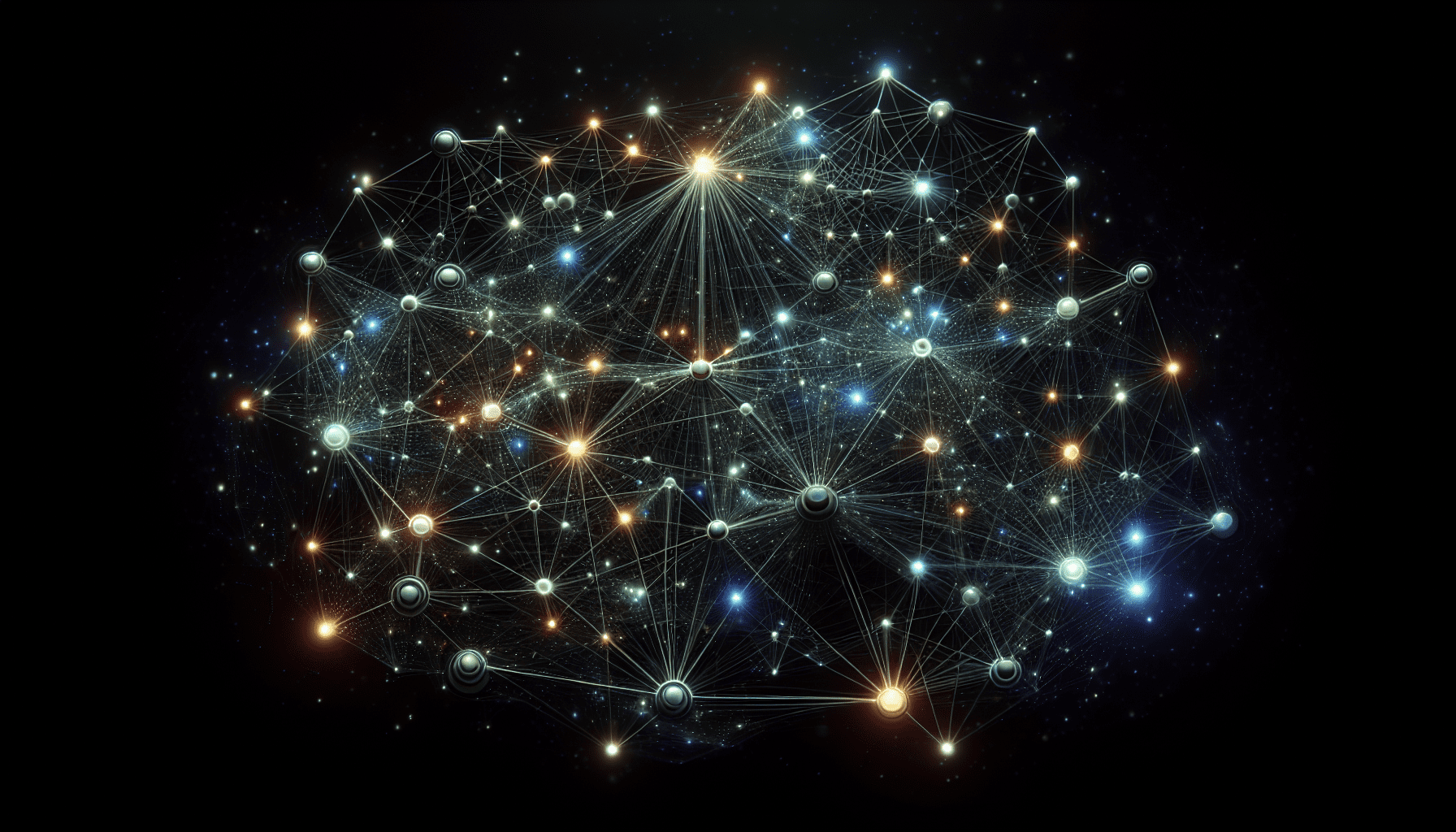
Generative AI, an exciting facet of artificial intelligence, can generate a wide array of content. From text to imagery, audio, and synthetic data, many generative AI models are transforming how we create and consume content. In addition, other generative AI models are being used to advance the field of artificial intelligence further. Based on machine learning, these techniques allow generative AI models to generate new data from existing datasets. But that’s just the beginning; we will examine the role of the foundational models.
The Role of Large Language Models
The advent of large language models such as GPT-4 has brought about a paradigm shift in the landscape of generative AI. These models have propelled generative AI towards more interactive and versatile applications across different domains.
One such application is Dall-E, a multimodal AI application that effectively identifies connections across various media types, including vision, text, and audio. It adeptly links the semantic meaning of words to visual elements, thereby exemplifying the expanding applications and capabilities of large language models.
The Emergence of Diffusion Models
Diffusion models have emerged as a cornerstone of generative AI, delivering high-quality outputs and offering adaptability across various use cases. These sophisticated deep neural networks iteratively learn the structure of data during training, transforming random noise into representative data samples. However, these models are not without their flaws.
One notable drawback is the slower reverse sampling process, which can act as a bottleneck when generating new data.
The Spectrum of Generative AI Applications
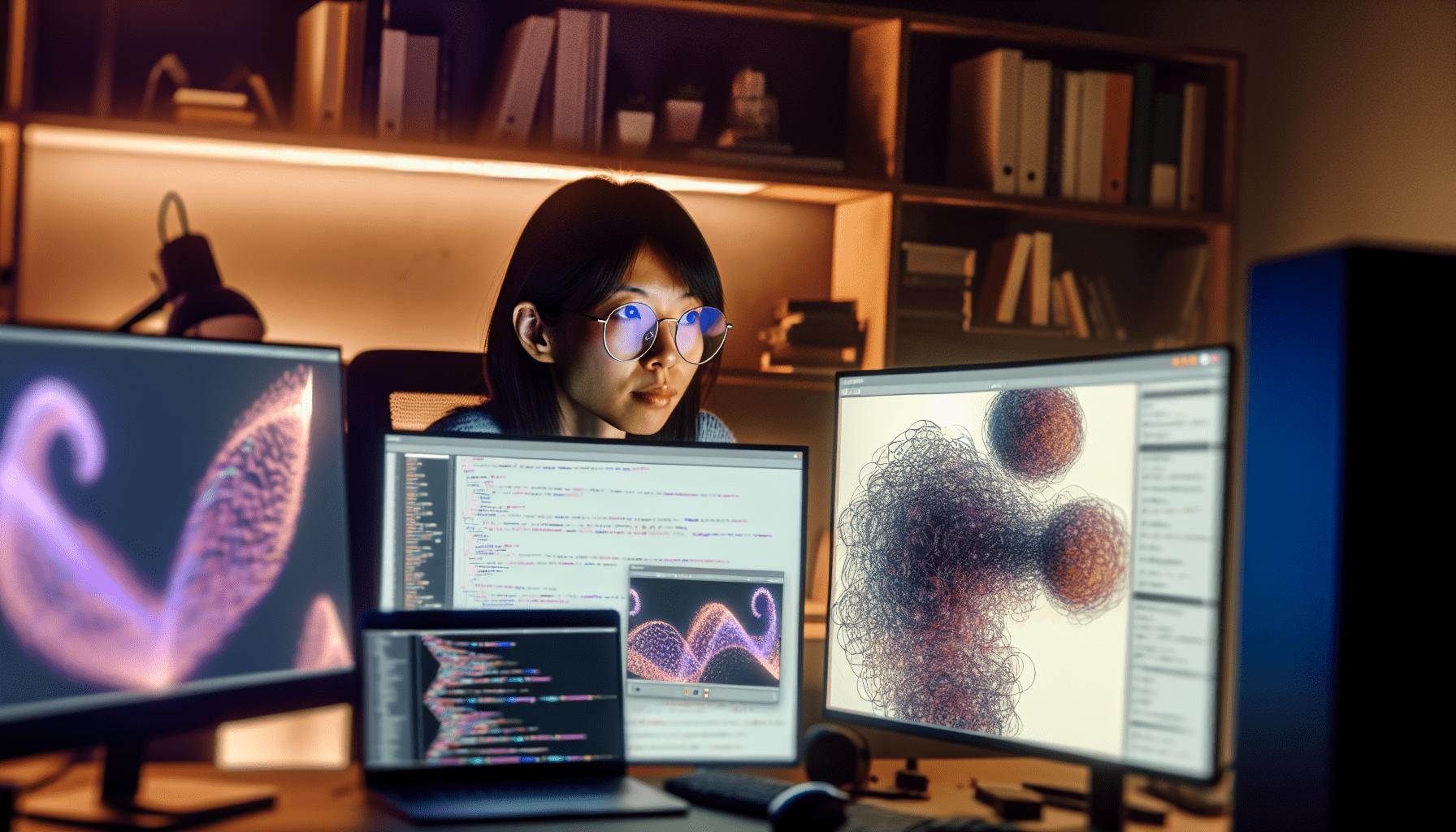
Generative AI’s potential extends far beyond creating engaging content. It has a broad spectrum of applications that span automated content creation, code generation, and even breakthroughs in the science and healthcare sectors, all using generative AI.
We will examine in detail how generative AI is transforming these sectors.
Content Creation Revolution
Generative AI is significantly transforming the content creation landscape. Be it text generation, image synthesis, or music composition, generative AI is automating these tasks and revolutionizing the way we create and consume content.
For instance, applications like Stable Diffusion can produce lifelike images based on textual descriptions. Similarly, generative AI is automating the process of music composition, paving the way for the creation of new music pieces with minimal human intervention.
Innovations in Code Generation
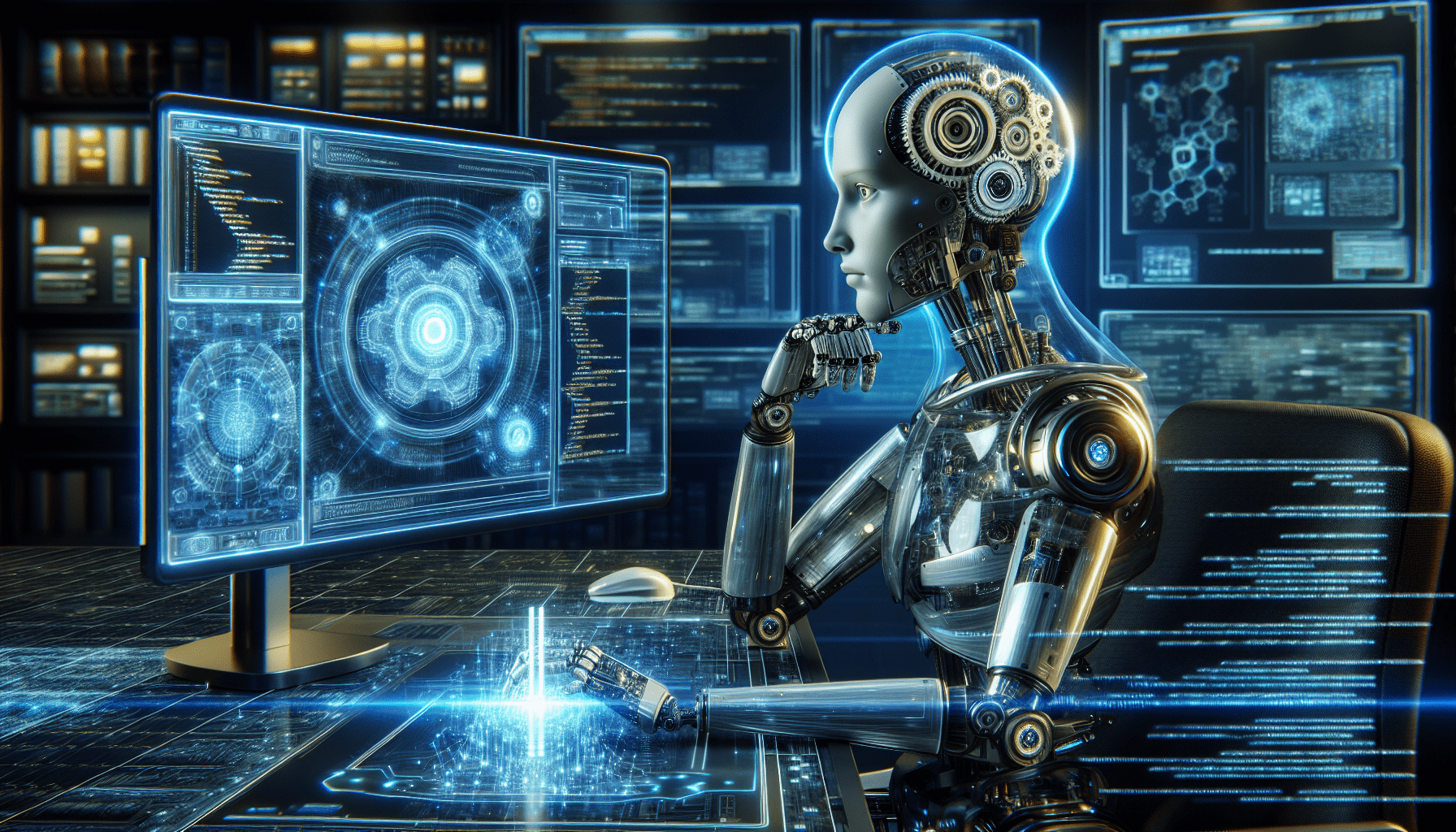
Generative AI is also making waves in the realm of software development. AI-driven code generation tools like OpenAI Codex, Copilot by Github, and ChatGPT by OpenAI are modernizing legacy code and streamlining the software development lifecycle. These AI tools analyze and refactor legacy code, generate tests through the analysis of existing code and data, and translate outdated programming languages into modern ones, thereby modernizing legacy systems.
Breakthroughs in Science and Healthcare
Generative AI is not limited to creative domains; it’s making significant contributions to science and healthcare as well. From expediting drug discovery to deciphering protein folding and enhancing medical imaging, generative AI is paving the way for new breakthroughs that could reshape these industries and enhance patient outcomes.
Navigating the Challenges and Risks of Generative AI
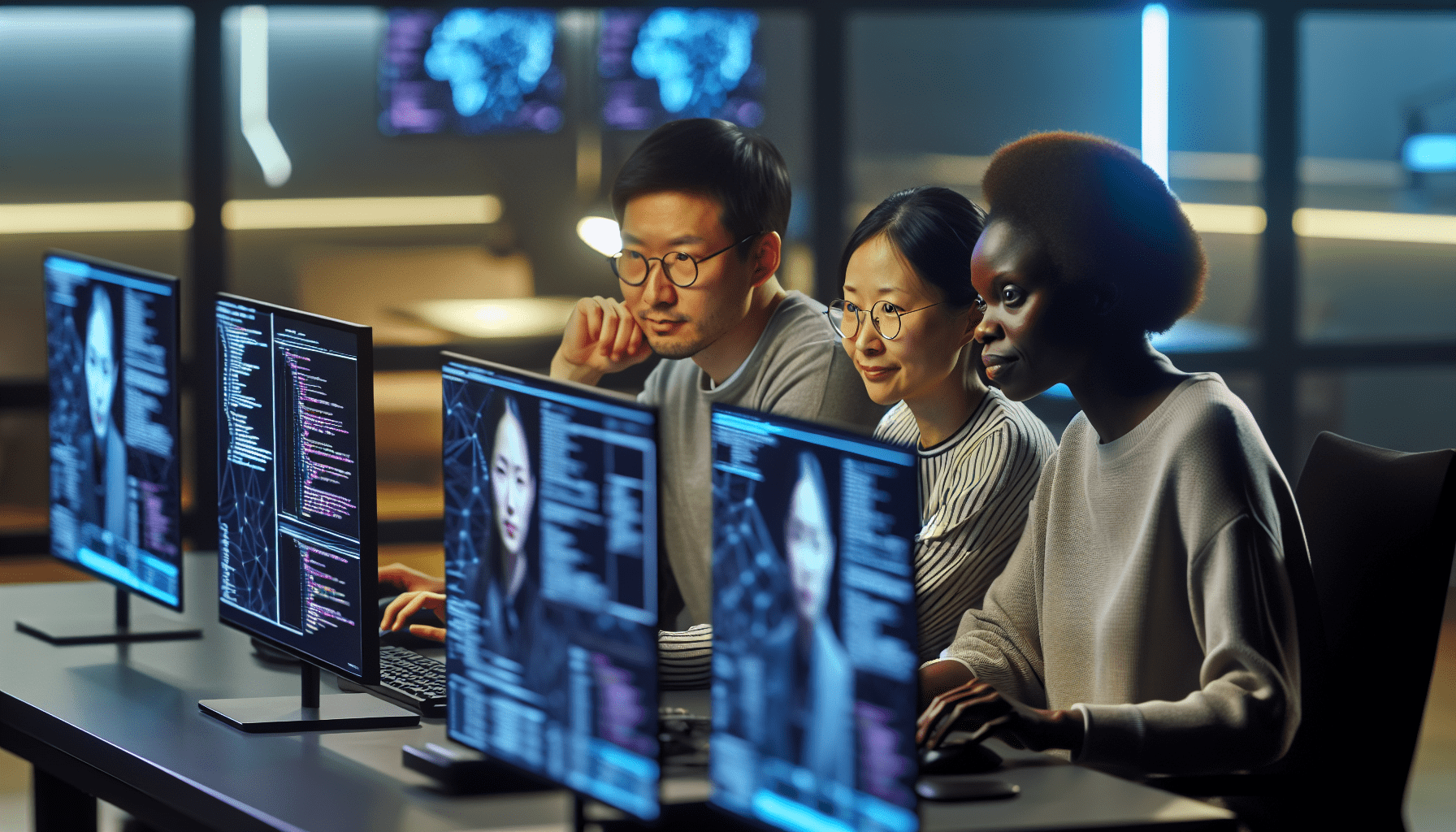
The journey of generative AI is not devoid of challenges and risks. Some of the key challenges and risks include:
-
Tackling bias and ensuring fairness in the generated content
-
Addressing security implications and protecting against malicious use of generative AI
-
Ensuring the sustainability of generative AI systems and minimizing their environmental impact
Navigating these challenges is an integral part of leveraging generative AI effectively.
We will further examine these challenges.
Mitigating Bias and Ensuring Fairness
Ensuring fairness and mitigating bias in generative AI is a challenge that requires meticulous model training and human supervision. For instance, image generation tools might produce images that reinforce gender or racial biases. To tackle this, researchers and developers are enhancing the training data and algorithms to minimize bias and ensure more diverse and equitable outputs.
This highlights the importance of human oversight in the process.
Security Implications and Deepfake Dangers
The security implications of generative AI are a cause for concern. Deepfakes, or artificially created media that appear real, represent a particular use of generative AI that emphasizes the creation of authentic and persuasive artificial media. The realistic and convincing nature of these deepfakes makes it easier to create false representations that are hard to detect, leading to potential misuse and abuse.
Sustainability and Resource Consumption
Generative AI technologies can be energy-intensive, leading to significant electricity usage and posing a sustainability issue. The computation required for creating and training AI models often entails significant energy use.
By optimizing resource consumption, not only can we reduce this impact, but we also pave the way for responsible and ethical development of such technologies for future scalability.
Best Practices in Utilizing Generative AI
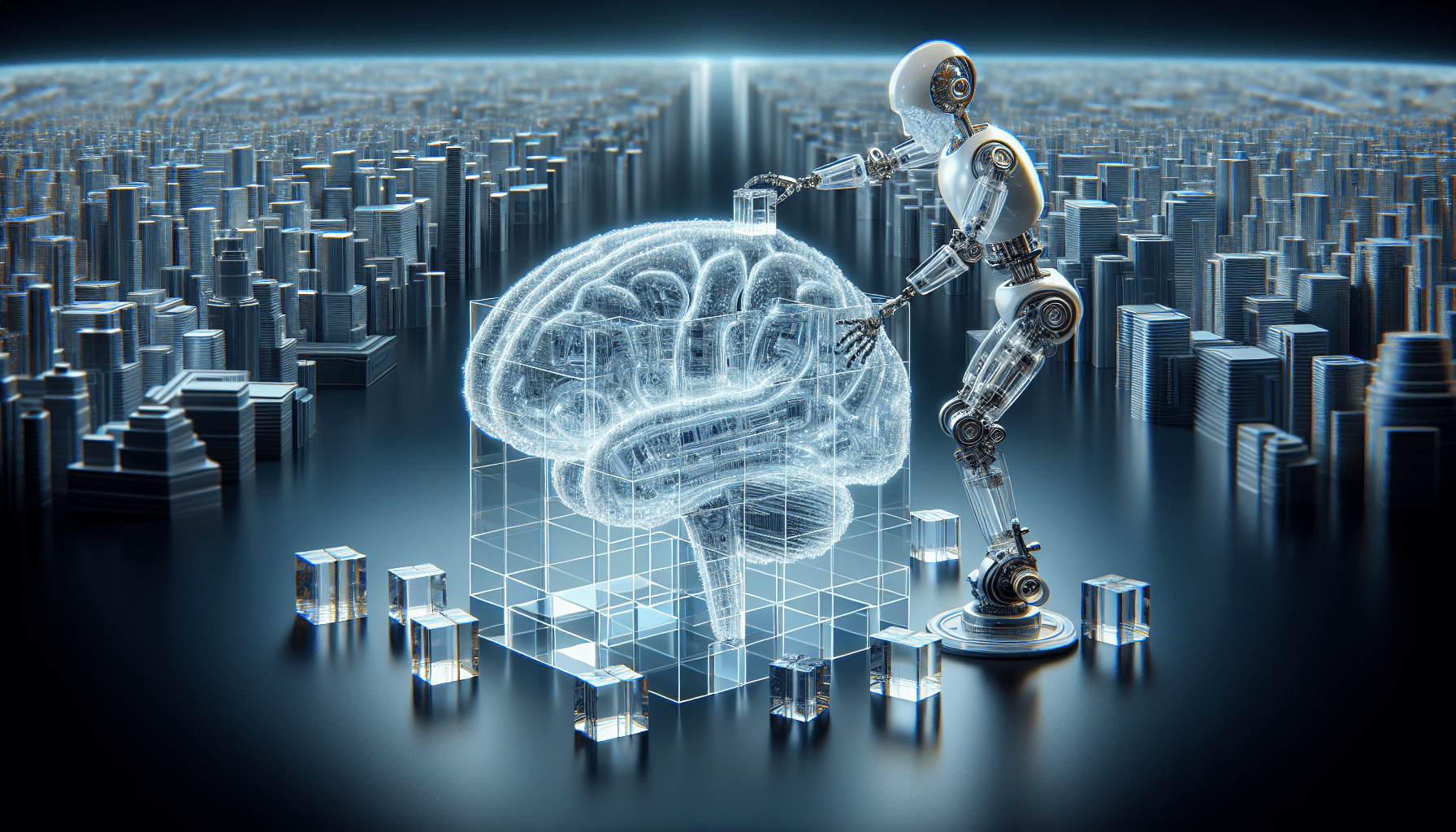
To harness the full potential of generative AI, it’s crucial to follow certain best practices. These include building trust through transparency, integrating human oversight, and addressing privacy and proprietary concerns.
We will further examine these practices.
Building Trust Through Transparency
Building trust in generative AI begins with transparency. It’s about being open and clear about the functionality of the AI algorithms and the rationale behind their decisions. From the use of Explainable Artificial Intelligence (XAI) techniques to the adoption of ‘model cards’, a variety of strategies can be employed to enhance transparency in the development of generative AI models.
Integrating Human Oversight
Human oversight is critical in validating generative AI outputs, ensuring accuracy, and mitigating potential risks. This encompasses strategies such as:
-
Fact-checking
-
Validation
-
Fine-tuning
-
Implementing guardrails to control biases and unpredictable outputs of generative AI models.
Data Privacy and Proprietary Concerns
Protecting data privacy and addressing intellectual property concerns are essential when implementing generative AI solutions. Proper adherence to a variety of data privacy regulations, such as the General Data Protection Regulation (GDPR), data protection laws, privacy policies, and cross-border data transfer restrictions, is crucial for upholding data privacy.
The Future Landscape of Generative AI
As we look to the future, generative AI holds immense potential for advancements in AI technology, transformation of industries, and philosophical considerations around human intelligence.
We will examine the potential future of generative artificial intelligence, including the impact of generative AI work.
Predictions for Advancements in AI Technology
Future advancements in AI technology will continue to enhance generative AI, leading to improvements in user experience, customization, and integration with various tools and workflows.
The evolution of AI will significantly improve customization through personalized product recommendations and smoother customer journeys, ultimately enhancing the overall user interaction with technology.
Generative AI’s Ability to Transform Industries
Generative AI has the potential to bring about significant changes across a range of industries by introducing new applications in:
-
3D modeling
-
Product design
-
Drug development
-
Digital twins
-
Supply chains
-
Business processes
It can also augment the capabilities of current tools such as grammar checkers, design tools, and training tools.
Philosophical Considerations: Approaching Human Intelligence?
The introduction of generative AI sparks discussions regarding the future of human cognition and the possible development of AI capabilities surpassing human abilities. It becomes necessary to reassess the significance and characteristics of human knowledge and proficiencies.
Furthermore, the potential development of AGI raises significant ethical and societal concerns that require effective management.
Leveraging Generative AI for Business Growth
Businesses can leverage generative AI for growth by increasing efficiency, driving creativity, and gaining a competitive advantage through AI adoption.
We will examine how generative AI can contribute to business growth.
Increasing Efficiency and Productivity
Generative AI has the capability to optimize workflows across various industries by automating tasks and processes, thereby enhancing operational efficiency. It can be applied in the following ways:
-
Automating customer service, marketing, and sales functions
-
Streamlining inventory and supply management
-
Enhancing data analysis and decision-making processes
By automating these essential functions, generative AI enables human resources to focus on more strategic initiatives, ultimately leading to improved efficiency and productivity in diverse industries.
Driving Creativity and Innovation
Generative AI contributes to driving creativity and innovation by:
-
Automating and augmenting creative processes
-
Enhancing the capabilities of designers, artists, and writers
-
Optimizing business processes for greater operational efficiency and growth.
Furthermore, generative AI can enhance creativity by offering fresh viewpoints and using generative AI to create innovative ideas.
Competitive Advantage Through AI Adoption
Adopting generative AI technologies can provide businesses with a competitive edge. By enhancing decision-making, reducing costs, and improving customer experiences, generative AI can provide valuable advantages that set businesses apart in the market. Indeed, research indicates that enterprises that implement generative AI may achieve cost reductions of up to 40% within the next five to seven years.
Summary
As we’ve explored, generative AI’s potential is vast and transformative. From content creation to scientific breakthroughs, it’s reshaping our world in ways we could only imagine a few years ago. However, with its potential come challenges: bias, security, and sustainability. Navigating these effectively requires transparency, human oversight, and a focus on data privacy. As we look to the future, the possibilities are endless. With continued advancements and careful implementation, generative AI promises to drive creativity, innovation, and growth in the years to come.
Frequently Asked Questions
What is generative AI vs normal AI?
Generative AI creates output based on its training data, while traditional AI identifies patterns from existing data.
Is GPT a generative AI?
Yes, GPT is a type of large language model and a prominent framework for generative artificial intelligence, used in natural language processing tasks.
How is generative AI transforming content creation?
Generative AI is transforming content creation by automating tasks such as text generation, image synthesis, and music composition. This advancement is revolutionizing the way content is produced and allowing for more efficient and innovative creation processes.
What challenges and risks are associated with generative AI?
Generative AI presents challenges and risks such as bias, security concerns related to deepfakes, and sustainability issues due to its energy-intensive nature. It is important to address these factors for responsible and ethical AI development.
What are the best practices for using generative AI?
When using generative AI, it is important to build trust through transparency, integrate human oversight, and address data privacy and proprietary concerns. Incorporating these practices can help ensure responsible and effective use of generative AI.