In the SaaS sector, the innovative edge offered by AI must be pursued with a vigilant sense of ethical responsibility. How can SaaS companies harness AI’s potential while ensuring ethical integrity? This article delves into the “ethical ai in saas balancing innovation and responsibility” address the ethical implications of AI in SaaS conundrum. We discuss the challenges and opportunities for SaaS providers in incorporating ethical principles into their AI-driven services, and outline ways to create AI that advances the industry and upholds the public trust.
Key Takeaways
-
Ethical AI concerns in SaaS include privacy, fairness, bias, transparency, and accountability, requiring a balance between technological innovation and ethical responsibility.
-
International frameworks and principles exist to guide the development and deployment of ethical AI, with strategies like implementing privacy-by-design and robust security measures to mitigate risks.
-
AI’s impact on job markets requires strategic response to job displacement through reskilling, while also fostering new skill development, with government policy playing a pivotal role.
The Intersection of AI Innovation and Ethical Responsibility in SaaS
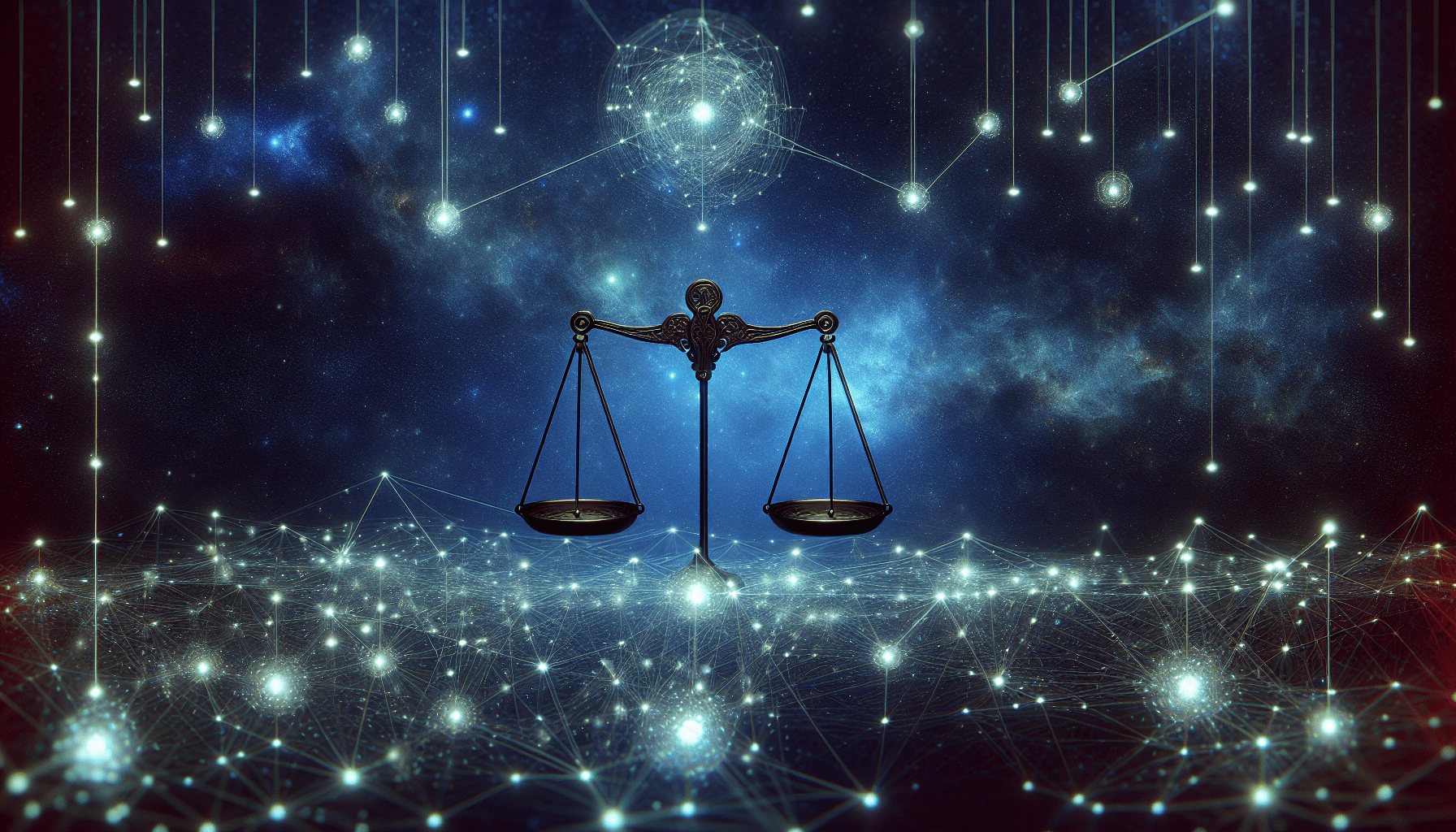
AI systems have transformed the SaaS landscape, streamlining processes, enhancing productivity, and opening up new avenues for innovation. Yet, the rapid proliferation of AI technologies has also raised valid ethical considerations. As these technologies interact more closely with humans, the ethical implications of AI become increasingly pronounced. Some of the key ethical concerns include:
-
Privacy concerns
-
Fairness issues
-
Bias in AI algorithms
-
Transparency and explainability of AI systems
-
Accountability for AI decisions
There is an urgent need to address these ethical concerns to ensure responsible and ethical use of AI.
The delicate dance of AI development involves striking a balance between innovation and ethical accountability. It involves navigating the tension between the drive for technological advancement and the need to ensure that the use of AI aligns with ethical standards, respects personal privacy, and avoids biased or unfair outcomes. This balance is not just about preventing harm but fostering technology that benefits society. Hence, incorporating ethical considerations in the design and deployment of AI systems is a significant facet of responsible AI development.
Pioneering with Purpose: The Role of Ethical Considerations in AI Development
In terms of securing ethical AI, we are not beginning from square one. There are already international governance frameworks for ethical AI development in place. For instance, the Carnegie Council and IEEE’s five-part framework, guidelines from countries such as Canada, EU, UK, and the USA, and UNESCO’s AI governance framework, all aim to ensure the responsible development and deployment of AI systems. These frameworks serve as a compass, guiding us towards a future where AI development is not just about pioneering with purpose but also doing so with a deep commitment to ethical considerations.
The principle of fairness in AI ethics, in particular, holds great significance. It is essential to mitigate the potential for biased outcomes in autonomous decision-making systems and to guarantee fair treatment for all user demographics. Considering ethical principles in AI development, such as fairness, helps in preventing the perpetuation of biases and unfair outcomes, thereby fostering the responsible development of AI-driven SaaS solutions.
Ensuring Ethical Deployment: Strategies to Mitigate Risks
With the increasing integration of AI systems into our daily lives, the need for ethical deployment turns into a vital concern. Ensuring transparency and accountability in the application of AI in decision-making processes is essential to maintain fairness. It also plays a key role in protecting individuals’ privacy rights. The emphasis is not solely on the ethical design of AI tools, but also their ethical use.
This is where the concept of privacy-by-design comes into play. It entails incorporating privacy considerations into the design and development of products and services right from the outset. To strike an appropriate equilibrium between AI and security/privacy considerations, we need to implement robust security measures, such as encryption and secure data handling practices, while adhering to privacy regulations. In a nutshell, any responsible AI development should feature privacy-by-design principles and be guided by ethical frameworks.
Crafting Ethical Principles for AI in SaaS
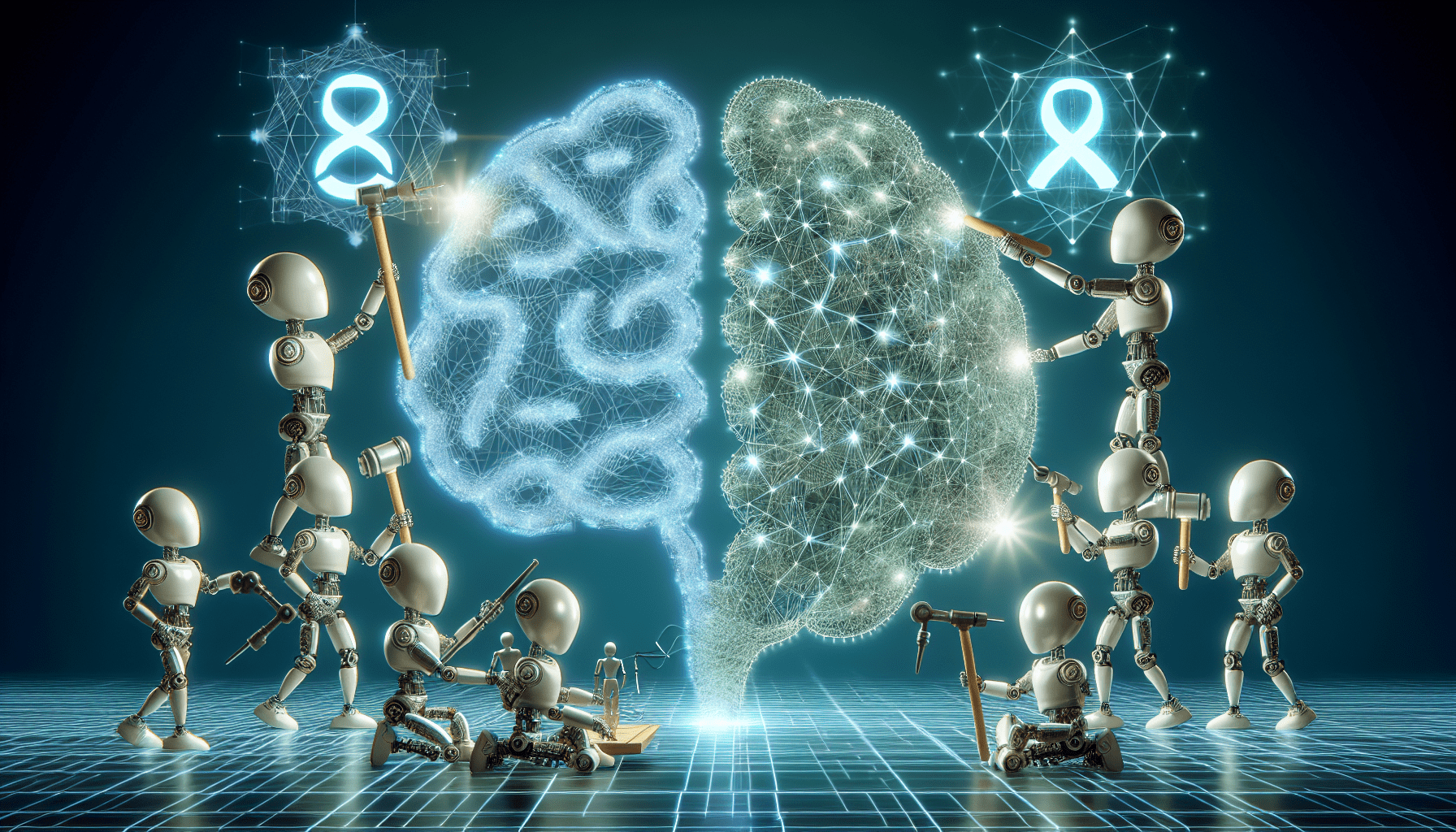
The journey towards ethical AI in SaaS begins with crafting clear and effective ethical principles that can guide AI development. The fundamental guiding principles for AI development in SaaS encompass:
-
Fairness
-
Transparency
-
Privacy and security
-
Accountability
-
A human-centered approach
These principles provide a structured framework for steering AI development, taking into account the effects on individuals, society, and the environment.
However, it’s worth noting that ethical AI principles don’t follow a ‘one-size-fits-all’ approach. Different industries have different values, priorities, and considerations, and these need to be reflected in their respective ethical AI principles. For instance, the healthcare industry may emphasize patient privacy and data security, while the financial industry may prioritize fairness and transparency in algorithmic decision-making. Adapting ethical AI principles to cater to the unique needs and values of each industry is crucial.
Harnessing AI Responsibly: A Framework for Ethical AI
Constructing a framework for ethical AI in SaaS involves the following components:
-
Ethical guidelines
-
AI governance model
-
AI ethics principles
-
Risk assessment
-
Reproducibility and explainability
-
Compliance with regulations
These components work together to provide a structure that enables us to harness AI responsibly, including natural language processing.
Key considerations for fairness in the development of an ethical AI framework in SaaS include:
-
Non-discrimination
-
Bias mitigation
-
Transparency
-
Equity
-
Privacy and data protection
Ensuring fairness helps prevent discriminatory practices and fosters an environment of trust and respect. Moreover, strategies for ensuring accountability, like governance, transparency and explainability, fairness, compliance, monitoring and evaluation, play a pivotal role in fostering responsible and ethical use of AI.
From Principles to Practice: Operationalizing AI Ethics
Operationalizing AI ethics in SaaS involves several steps:
-
Identify the current infrastructure to support a data and AI ethics program.
-
Establish a program that aligns with organizational values.
-
Formulate a code of ethics for AI development.
-
Implement guidelines, policies, and procedures that promote ethical AI.
-
Maintain human oversight throughout the AI development process.
By following these steps, organizations can ensure that ethical considerations are integrated into their AI practices in SaaS.
Methods for translating AI ethics principles into practical guidelines include:
-
Addressing misconceptions
-
Adopting high-level principles
-
Developing measurable guidelines
-
Ensuring implementation aligns with commitments
-
Bridging the gap through digital cooperation
-
Embedding principles into AI tools
-
Promoting continuous ethical reflection
The aim is to prioritize ethical considerations, ensuring they are not merely theoretical concepts, but are deeply woven into the fabric of AI development processes.
AI Technologies and Data Privacy: Navigating the Tightrope
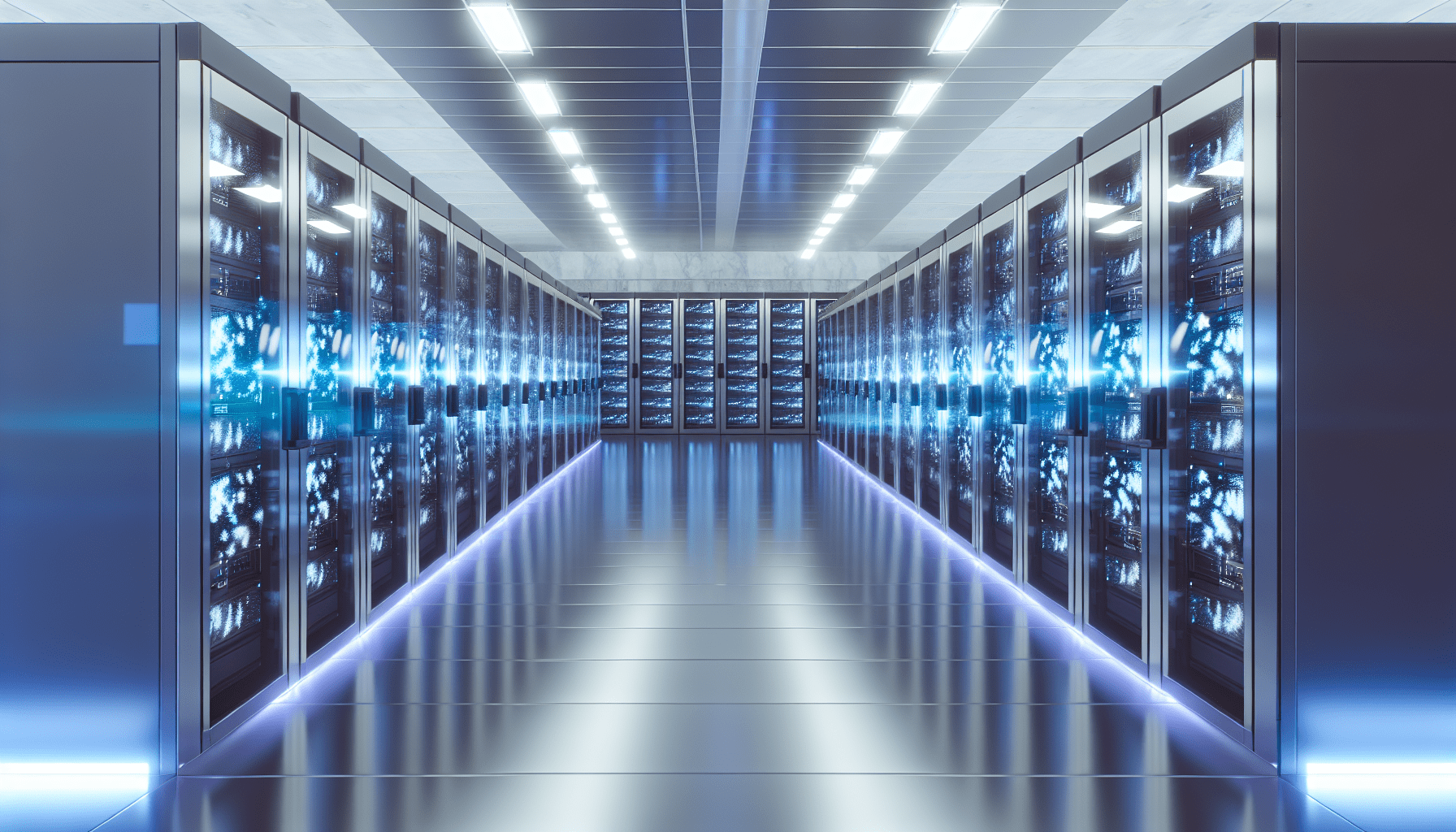
As AI technologies continue to evolve and proliferate, data privacy has emerged as a significant concern. Protecting sensitive data in AI-driven SaaS solutions requires rigorous security measures, including:
-
Implementing robust authentication methods
-
Employing data encryption techniques
-
Regularly conducting security audits and reviews
-
Maintaining data resilience to minimize data loss.
In data collection for AI, trust and transparency hold equal significance. Companies can establish trust by openly disclosing their data collection methods, adhering to stringent data protection regulations, and prioritizing the protection of personal information. In an era where data is the new oil, the ethical collection and usage of data is of utmost importance.
Protecting Sensitive Data: The Imperative of Robust Security Measures
Despite the immense benefits of AI-driven SaaS solutions, they are not devoid of vulnerabilities. These vulnerabilities can range from:
-
model poisoning
-
data privacy breaches
-
data tampering
-
insider threats
To address these vulnerabilities and safeguard against data breaches and the compromise of sensitive information, it is imperative to implement robust security measures.
Categories of sensitive data in AI-driven SaaS solutions usually include personal identifying information, financial information, and personal health information that require strict protection measures. The role of encryption in safeguarding sensitive data cannot be overstated – it provides secure storage and transmission, ensuring that only authorized entities can access the encrypted data. Alongside encryption, the use of behavioral biometrics for user verification, the application of AI for anomaly detection and endpoint protection, and the utilization of predictive threat intelligence to anticipate potential security incidents are all key security measures that can protect sensitive data in AI-driven SaaS solutions.
Transparency and Trust: Prioritizing Ethical Considerations in Data Collection
Transparency is a cornerstone of ethical data collection. Companies can foster trust and transparency by clearly communicating their data practices to users and adhering to privacy-by-design principles. Furthermore, responsible management of the data lifecycle and enhancing accountability and trust with customers are also crucial aspects of ethical data collection.
For SaaS companies, building a trustful relationship with customers concerning data usage in AI applications is critically important. It plays a critical role in fostering a strong and ethical relationship with customers, ensuring responsible handling of their data.
To achieve this trust, here are some methods for utilizing AI to improve data privacy and guarantee ethical data collection:
-
Implement privacy-preserving AI technology
-
Have transparent data collection policies
-
Conduct ethical data analysis
-
Adhere to established AI ethics principles
Transparency in data practices and demonstrating accountability are key to achieving this trust.
The Impact of AI on Job Markets: A Dual-Edged Sword
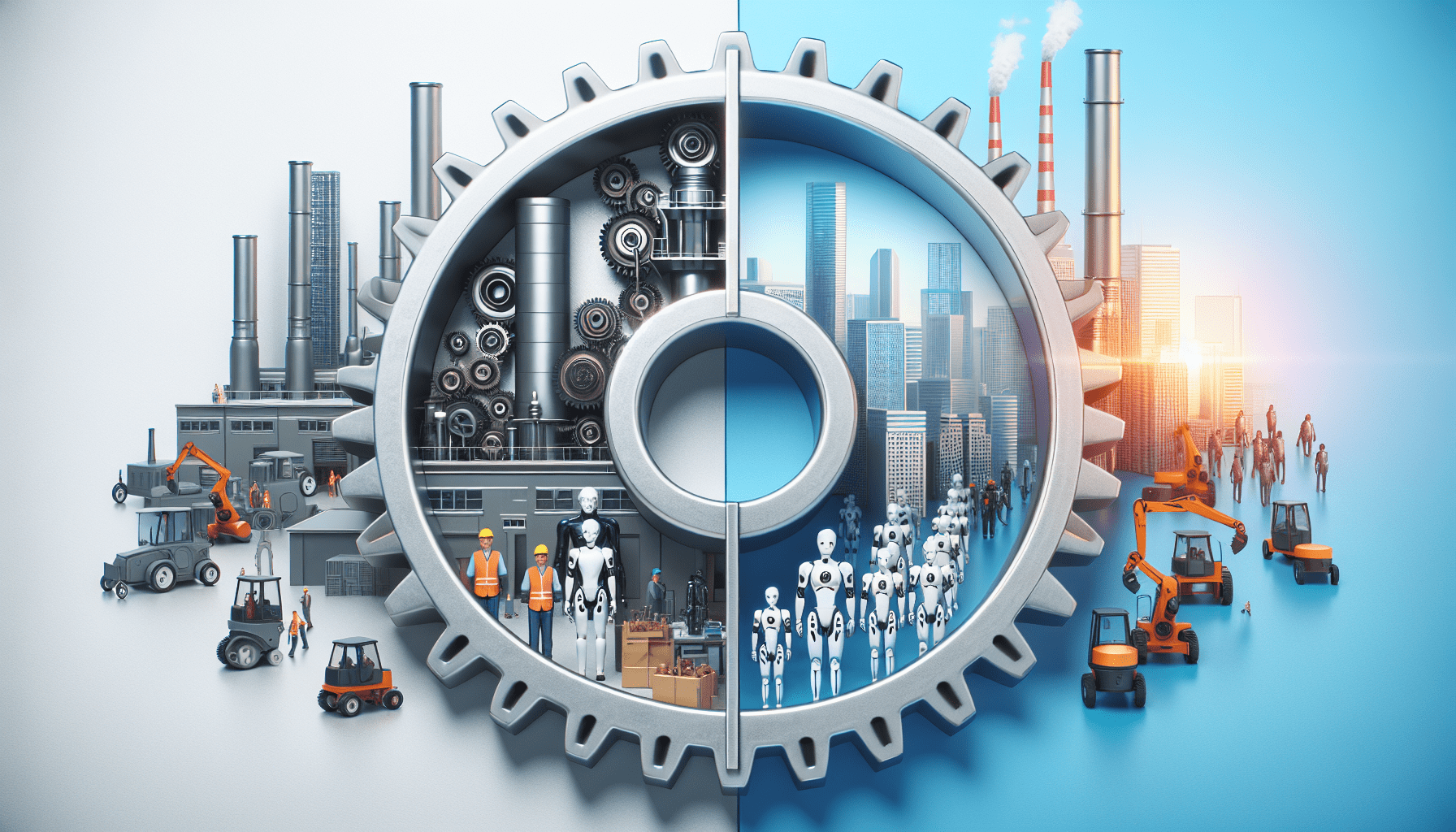
AI technologies have significantly influenced job markets. Certain sectors, particularly those involving repetitive tasks or heavy data processing, like tech jobs and media jobs, are particularly vulnerable to AI technologies. However, it’s not all doom and gloom. On the flip side, AI is also contributing to the creation of new job opportunities by facilitating the emergence of roles such as:
-
deep learning engineers
-
AI chatbot developers
-
data annotators
-
AI-powered digital assistants
Indeed, the increasing prevalence of AI-driven roles is causing a significant shift in the job market. This not only involves the evolution or obsolescence of traditional roles but also the rise of new roles that demand a greater understanding of AI and related technologies. As AI continues to advance, professionals will need to adopt a skills-first approach to effectively navigate and excel in this evolving landscape.
Navigating Job Displacement with Compassion and Strategy
The rise of AI, while opening up exciting opportunities, also triggers job displacement in some sectors. Addressing this challenge requires compassionate and strategic approaches, such as reskilling programs and support for affected workers. Workforce reskilling and upskilling programs are crucial strategies for assisting workers affected by job displacement due to AI technologies. These programs equip individuals with the necessary skills for new roles resulting from advancements in AI.
Government policies hold a key role in mitigating job displacement caused by AI. They are instrumental in:
-
Developing strategies
-
Providing income support
-
Promoting job creation
-
Safeguarding workers’ rights during the transition of industries towards AI reliance
Indeed, reskilling programs have demonstrated effectiveness in mitigating unemployment resulting from AI advancements. They facilitate the adaptation of workers to the changing job landscape and promote the development of new, sought-after skills.
Cultivating New Skills: The Emergence of AI-Driven Opportunities
AI-driven opportunities are revolutionizing the workforce and fostering the development of new skills. From data science and AI ethics to machine learning operations and cybersecurity, AI is opening up a world of possibilities. To prepare the current workforce for these new AI-enabled roles, the following steps are crucial:
-
Implement upskilling and reskilling programs
-
Promote a culture of lifelong learning
-
Integrate ethical AI training into the curriculum of educational institutions
Transitioning to an AI-empowered job market comes with its own set of challenges. These include:
-
The potential risk of job displacement in specific sectors
-
The necessity for ongoing learning to remain abreast of new technologies
-
The imperative to ensure equitable access to education and opportunities for all individuals
As we navigate this changing landscape, it’s important to remember that with every challenge comes an opportunity – an opportunity to learn, grow, and adapt.
AI Bias and Discrimination: A Call for Action

While traversing the AI revolution, we must address the glaring issue at hand – AI bias and discrimination. AI bias is a result of several factors, including biased training data, decisions made in algorithmic design, and pre-existing biases in the data created by humans. Biased AI systems can have serious implications, such as reinforcing stereotypes, restricting opportunities for specific groups, and perpetuating social inequalities. This can lead to unfairness and discrimination in areas such as hiring, lending, and criminal justice.
Methods for identifying bias in AI include analyzing the disparate impact on different groups, conducting fairness audits, and utilizing interpretability tools. To address AI bias, it is recommended to improve data collection, implement algorithmic fairness techniques, and ensure an inclusive team composition. As we strive for technological advancement, we must not lose sight of our commitment to uphold fairness and equality.
Identifying and Mitigating Bias in Machine Learning Algorithms
Machine learning algorithms often harbor a complex issue of bias, originating from biased training data, assumptions, and human biases intertwined within. This can lead to compromised purpose of machine learning, disparate impact on certain groups of people, unfair and discriminatory outcomes, loss of customer trust and revenue, and the potential for producing unjust results.
Addressing bias in machine learning algorithms is a multi-faceted task. It involves:
-
Identifying potential sources of bias
-
Establishing guidelines and rules for its elimination
-
Using pre-processing algorithms like relabelling and perturbation
-
Employing sampling techniques
-
Implementing in-processing algorithms to mitigate bias
-
Ensuring transparency about the chosen training datasets and mathematical processes used
Furthermore, using a diverse training dataset can help reduce bias, and algorithmic bias can be detected and mitigated through best practices and policies.
Building Inclusivity: The Role of Diverse Teams in AI Development
Diverse teams in AI development offer a unique advantage. By bringing together individuals from varied backgrounds, cultures, and demographics, diverse teams can:
-
effectively identify biases
-
develop solutions to mitigate them
-
provide insights into the potential impact of AI systems on different communities
-
ensure fairness and inclusivity for all users.
Advocating diversity in AI development teams goes beyond fairness – it’s about enhancing effectiveness too. Diverse teams can bring:
-
Heightened creativity and problem-solving
-
Reduced biases
-
Improved detection of bias
-
Increased innovation
-
Elevated engagement and productivity
Implementing inclusive hiring practices, ensuring equal opportunities, fostering a culture of inclusion, addressing biases in AI algorithms, and collaborating with organizations focused on increasing diversity in AI – all these strategies can help promote diversity in AI development teams.
Facilitating Ethical AI Through Regulatory Frameworks
AI regulation has embarked on a journey characterized by constant development. From the creation of the Logic Theorist in 1955 to the implementation of the AI Act in 2022, AI regulation has come a long way. Although these regulations set vital guidelines and standards for ethical AI usage, they may impose constraints that could potentially slow down innovation within the SaaS industry, particularly for AI system development.
Regulatory frameworks play a crucial role in managing privacy issues, ensuring accountability in AI systems, and navigating the fast pace of AI advancements. Significant regulations overseeing the use of AI technology include the General Data Protection Regulation (GDPR), the California Consumer Privacy Act (CCPA), and the Fair Credit Reporting Act (FCRA). These regulations are aimed at ensuring data privacy and fair usage of AI technology. Mitigating risks and fostering trust in AI technologies are crucial, requiring responsible governance, regulatory frameworks, and ongoing ethical evaluations. These measures are important to ensure the ethical and safe use of AI technologies.
The Evolution of AI Regulations: Balancing Innovation and Responsibility
The evolution of AI regulations involves harmonizing innovation with responsibility. It’s about ensuring that we harness the benefits of AI while also addressing the ethical considerations that come with it. As AI regulations continue to evolve, they present both opportunities and challenges for the SaaS industry. On one hand, they establish guidelines and standards that promote ethical AI usage. On the other hand, they can impose limitations that potentially hinder innovation.
Navigating these challenges requires a deep understanding of the evolving regulatory landscape. The widespread adoption of AI has led to the development of new regulations addressing concerns such as data privacy, algorithmic bias, and the necessity for transparency in AI systems. It’s a complex and ever-changing terrain, but one that holds the key to the responsible and ethical use of AI in SaaS.
Collaborating for Compliance: Industry and Government Partnerships
The ethical implications of AI cannot be addressed by a single entity alone. It requires a collaborative effort from various stakeholders, including:
-
Technology companies
-
Academic institutions
-
Civil society groups
-
Government entities
Partnerships between industry and government play a crucial role in creating ethical guidelines and setting standards for responsible AI implementation.
Such collaborations have already led to significant strides in AI regulation. For instance, the Partnership on AI (PAI) – a global coalition dedicated to promoting collaboration and ethical AI governance practices – is a shining example of what can be achieved when different stakeholders come together with a common goal. Such partnerships aid in building a sustainable and ethical approach towards AI regulation and guarantee adherence to ethical AI standards in SaaS.
Summary
As we stand on the cusp of a new era of AI-powered SaaS, it’s clear that ethical considerations must be at the forefront of our journey. From the intersection of AI innovation and ethical responsibility to the impact of AI on job markets and the urgent need to address AI bias and discrimination, the path to ethical AI in SaaS is a challenging yet rewarding journey. It requires careful navigation, strategic foresight, and a deep commitment to fairness, transparency, and inclusivity. As we embrace the immense potential of AI, let’s also pledge to uphold ethical AI practices – for the sake of our present and for the promise of a better future.
Frequently Asked Questions
What are the ethical implications of AI technology?
The ethical implications of AI technology include the perpetuation of societal biases, leading to unfair and discriminatory outcomes in crucial areas such as hiring, lending, criminal justice, and resource allocation. These biases can become ingrained in AI algorithms, contributing to discrimination and lack of transparency in decision-making.
What is AI ethics and responsible AI?
AI ethics refers to the moral principles and guidelines governing the use and development of artificial intelligence, while responsible AI involves the practical implementation and usage of AI in a manner that aligns with ethical values and societal benefits. Both terms are crucial in ensuring the positive impact of AI on individuals, businesses, and society.
How can AI systems be designed to act in an ethical and responsible manner?
AI systems can be designed to act in an ethical and responsible manner by prioritizing transparency, fairness, and algorithmic ethics, which are crucial for ensuring accountability and trustworthiness.
What are the strategies for addressing bias in machine learning algorithms?
To address bias in machine learning algorithms, it’s important to identify potential sources of bias, establish guidelines for its elimination, use pre-processing algorithms like relabelling and perturbation, and employ sampling techniques. By implementing these strategies, we can work towards reducing bias in machine learning models.
How can we protect sensitive data in AI-driven SaaS solutions?
To protect sensitive data in AI-driven SaaS solutions, you can implement robust authentication methods, employ data encryption techniques, regularly conduct security audits and reviews, and maintain data resilience to minimize data loss.