Integrating AI into your business operations can be a game-changer. Are you looking to streamline workflows, automate routine tasks, and unlock actionable insights from your data? This article serves as your roadmap to machine learning integration, highlighting critical steps, addressing common challenges, and illustrating the transformative impact with real-world examples. Dive in to turn the promise of machine learning into tangible results for your business.
Key Takeaways
-
Generative AI and Large Language Models enhance business efficiency by automating repetitive tasks, streamlining workflows, and extracting insights from data, which allows employees to focus on higher-value activities.
-
Implementing Generative AI in business requires identifying clear objectives, selecting the right tools and technologies, and an iterative process of building, training, evaluating, and optimizing the AI models to ensure they align with business goals.
-
While Generative AI and Large Language Models offer transformative benefits across various sectors, businesses must navigate challenges such as data quality, privacy, security, and ethical considerations to achieve successful integration.
The Power of Generative AI and Large Language Models
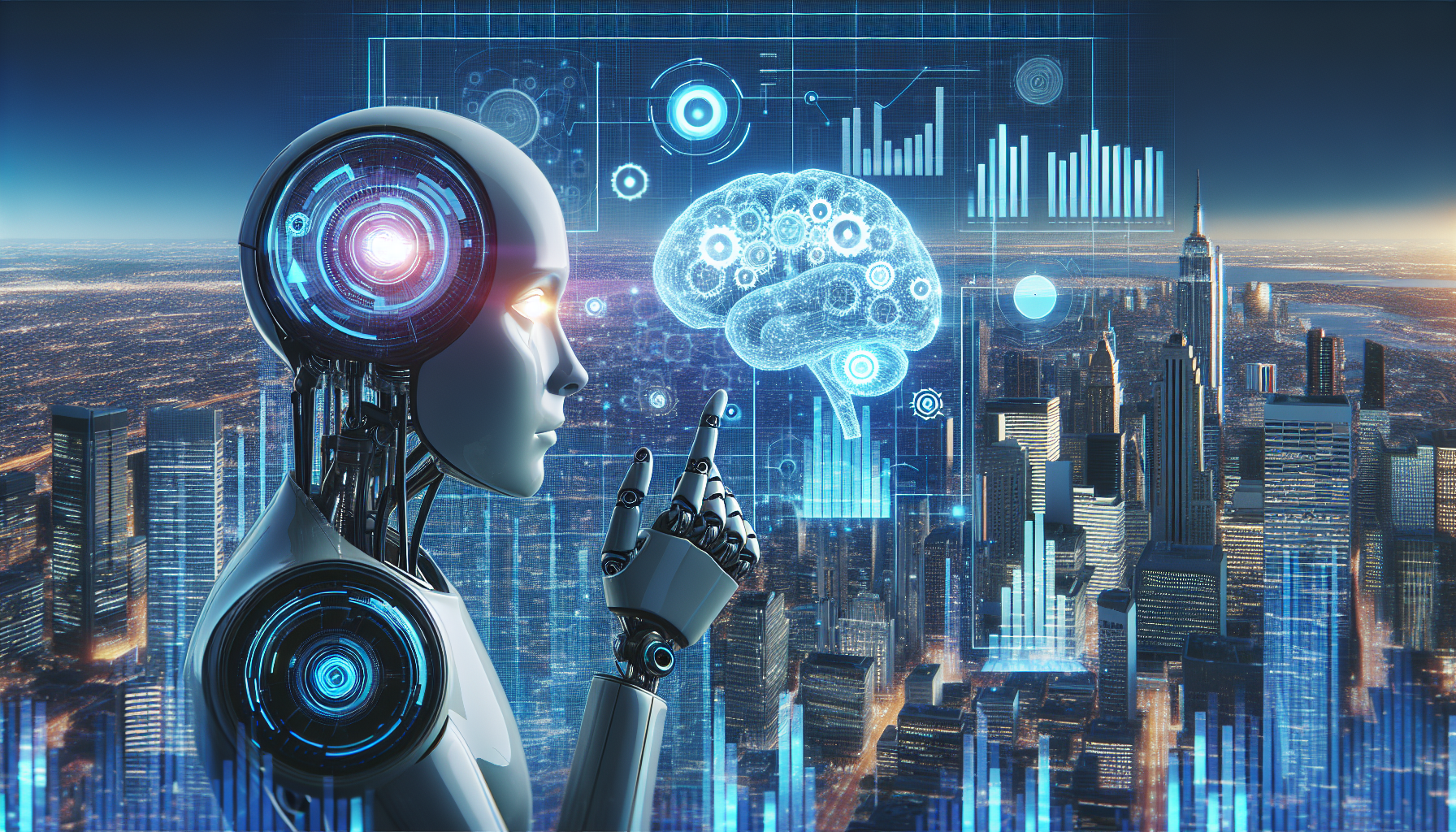
Generative AI, machine learning algorithms, and Large Language Models have revolutionized businesses’ operations. These technologies automate mundane tasks, allowing employees to focus on value-add activities, thus causing a surge in labor productivity. The result? Enhanced efficiency and a significant impact on business sectors, including predictive and data analytics too.
Enhancing Automation
Generative AI, defined as artificial intelligence capable of producing original content through analyzing existing data, has emerged as a game-changer in business operations. It offers several benefits, including:
-
Automating repetitive duties
-
Reducing the need for human intervention
-
Streamlining workflows
-
Increasing efficiency and productivity
The automation achieved through Generative AI offers several benefits, including:
-
Saving time and effort
-
Liberating resources for strategic and value-adding endeavors, such as AI in SaaS
-
A significant boost in productivity
-
An enhancement in overall operational efficiency
Data Analysis and Insights
Large language models, such as machine learning models, have brought a paradigm shift in data analysis and natural language processing. These models comprehend complex sentences, assess user intent, and produce new content, enabling software engineers to extract valuable insights from vast datasets.
AI further enhances this process by:
-
Identifying trends, patterns, and anomalies in large datasets
-
Providing a deeper understanding of operations, customer behavior, and market dynamics
-
Enabling businesses to make more informed, data-driven decisions
Personalized Customer Experiences
In today’s customer-centric business landscape, AI is pivotal in creating personalized customer experiences. AI-based systems analyze user behavior and offer tailored recommendations, saving users time, reducing decision fatigue, and increasing satisfaction. This ultimately enhances engagement, conversion rates and overall satisfaction.
Implementing Generative AI and Large Language Models: Key Steps
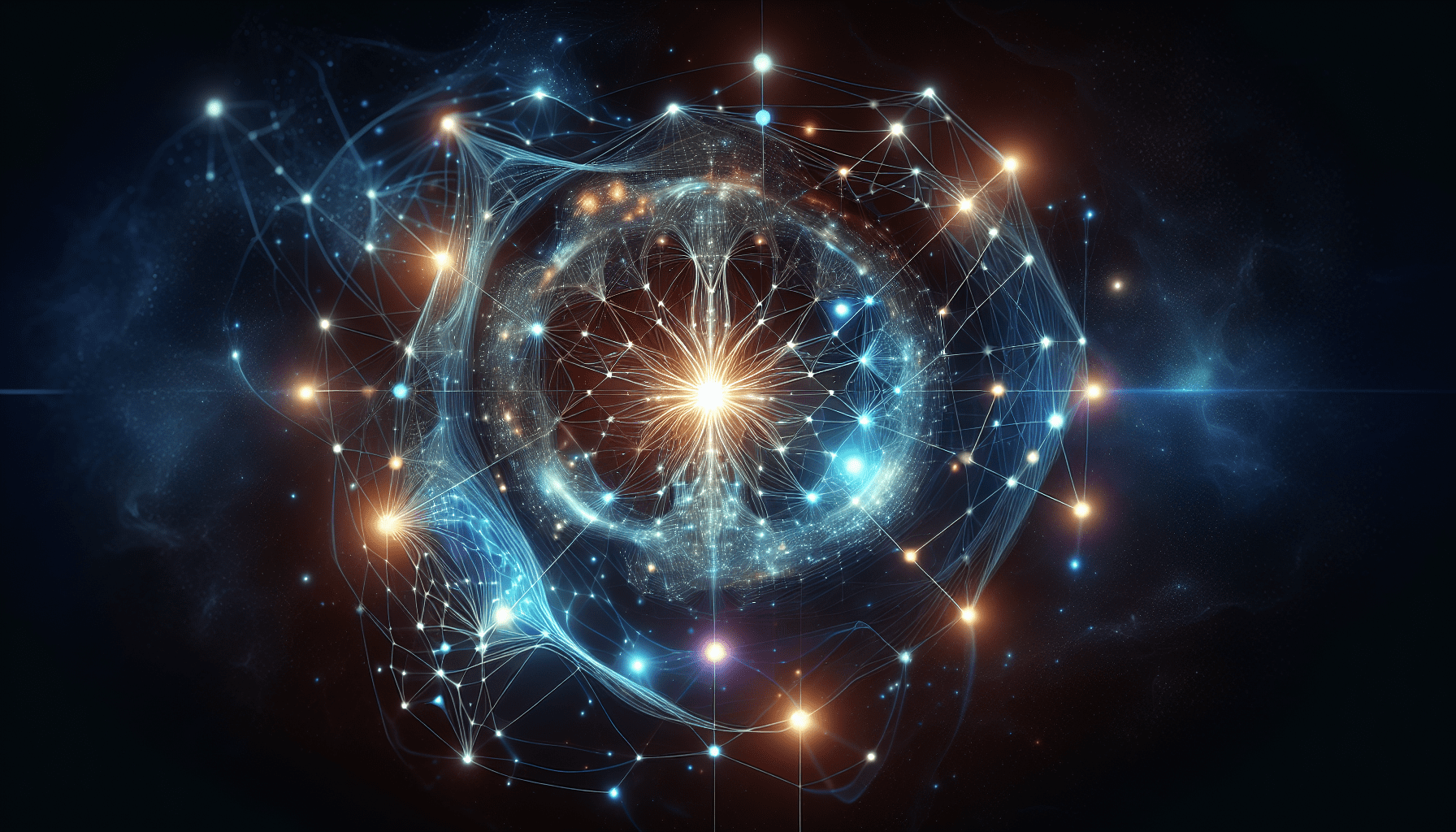
Implementing Generative AI and Large Language Models in a business involves several key steps to ensure success. Each step, from identifying business objectives to selecting the right tools and technologies, building and training models, and evaluating and optimizing performance, plays a crucial role in harnessing the true potential of these technologies.
Identifying Business Objectives
The first step towards successful AI integration is identifying clear business objectives. Some examples of business objectives that can be achieved through AI integration include the following:
-
Enhancing creativity and content generation
-
Improving customer interactions
-
Supporting marketing and sales efforts
-
Optimizing data summarization and synthesis
Understanding your business objectives aligns AI initiatives with your overarching goals, ensuring maximum return on investment.
Selecting the Right Tools and Technologies
Selecting the right tools and technologies is a critical step in AI integration. Here are some factors to consider:
-
Evaluate your business needs and compare features of different AI tools.
-
Assess the costs associated with implementing and maintaining the AI tool.
-
Consider the scalability of the tool and its ability to integrate with your current systems.
-
Ensure that the selected AI tool can grow with your business.
Keeping these factors in mind will ensure that the chosen tools will integrate smoothly into your existing systems and meet your business needs, making them an excellent option.
Building and Training Generative AI and Large Language Models
Building and training Generative AI and Large Language Models requires careful planning and execution. The process begins with collecting a substantial amount of textual data, preprocessing the data, and subsequently training the language model using the collected and preprocessed data.
Choosing the right generative model architecture is also a critical step. Factors to consider include:
-
Model quality
-
Infrastructure cost
-
Application latency
-
‘Out-of-the-box’ task performance
-
Inference speed
-
Overall Cost
The iterative refinement of prompts, including user feedback and adjusting hyperparameters, also plays a significant role in improving the efficiency and performance of large language models.
Evaluating and Optimizing Performance
Evaluating and optimizing performance is an ongoing process in AI integration. Adherence to evaluation protocols designed for text generation and large language models, coupled with consistent refinement of the AI algorithm, can improve the performance and usability of these technologies.
Regular feedback loops are crucial in this process, enabling continuous refinement of models based on incoming data.
Overcoming Challenges in Generative AI and Large Language Model Integration
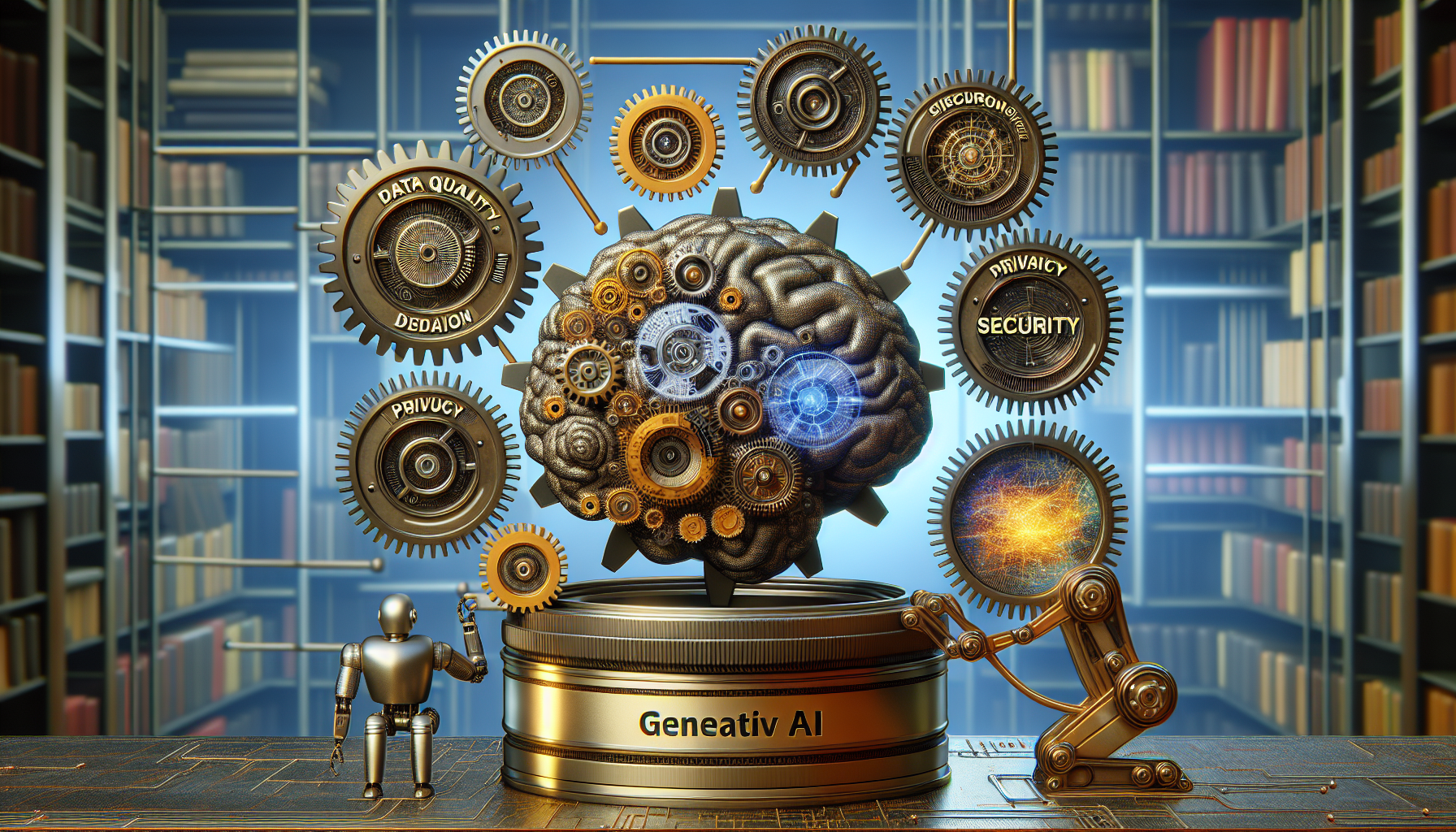
Like any technology, implementing Generative AI and Large Language Models comes with its own set of challenges. Addressing data quality and preparation issues, ensuring privacy and security, and considering ethical implications are key challenges businesses must navigate to integrate these technologies successfully.
Data Quality and Preparation
Ensuring high-quality data is critical for the success of AI initiatives. Comprehensive data cleaning and pre-processing are required to mitigate challenges related to subpar data quality and insufficient or erroneous information, which can introduce noise into model training.
Ensuring Privacy and Security
When integrating AI into their operations, businesses must prioritize privacy and security. To achieve this, there are some essential steps business leaders say they need to take to implement robust data protection measures.
-
Gather only essential data to prevent the collection of unnecessary personal information.
-
Set clear data retention policies to ensure that data is not kept for longer than necessary.
-
Obtain informed consent from the individuals whose data is being collected.
-
Incorporate secure measures to protect the data from unauthorized access or theft.
By following these crucial steps, businesses can establish a strong foundation for privacy and security in AI integration, and safeguard the sensitive information of their clients and customers.
Ethical Considerations
When it comes to leveraging AI for businesses, ethical considerations cannot be overlooked. To ensure that AI technologies are developed and deployed in a responsible and fair manner, businesses need to take various measures. These measures include addressing potential biases in AI models, advocating for fairness, and ensuring meticulous data handling. Additionally, businesses need to establish clear ethical standards for AI development and deployment, which prioritize transparency and accountability. By doing so, businesses can leverage the benefits of AI while ensuring that it is used in a responsible and ethical way.
Real-World Examples: Generative AI and Large Language Model Integration in Action
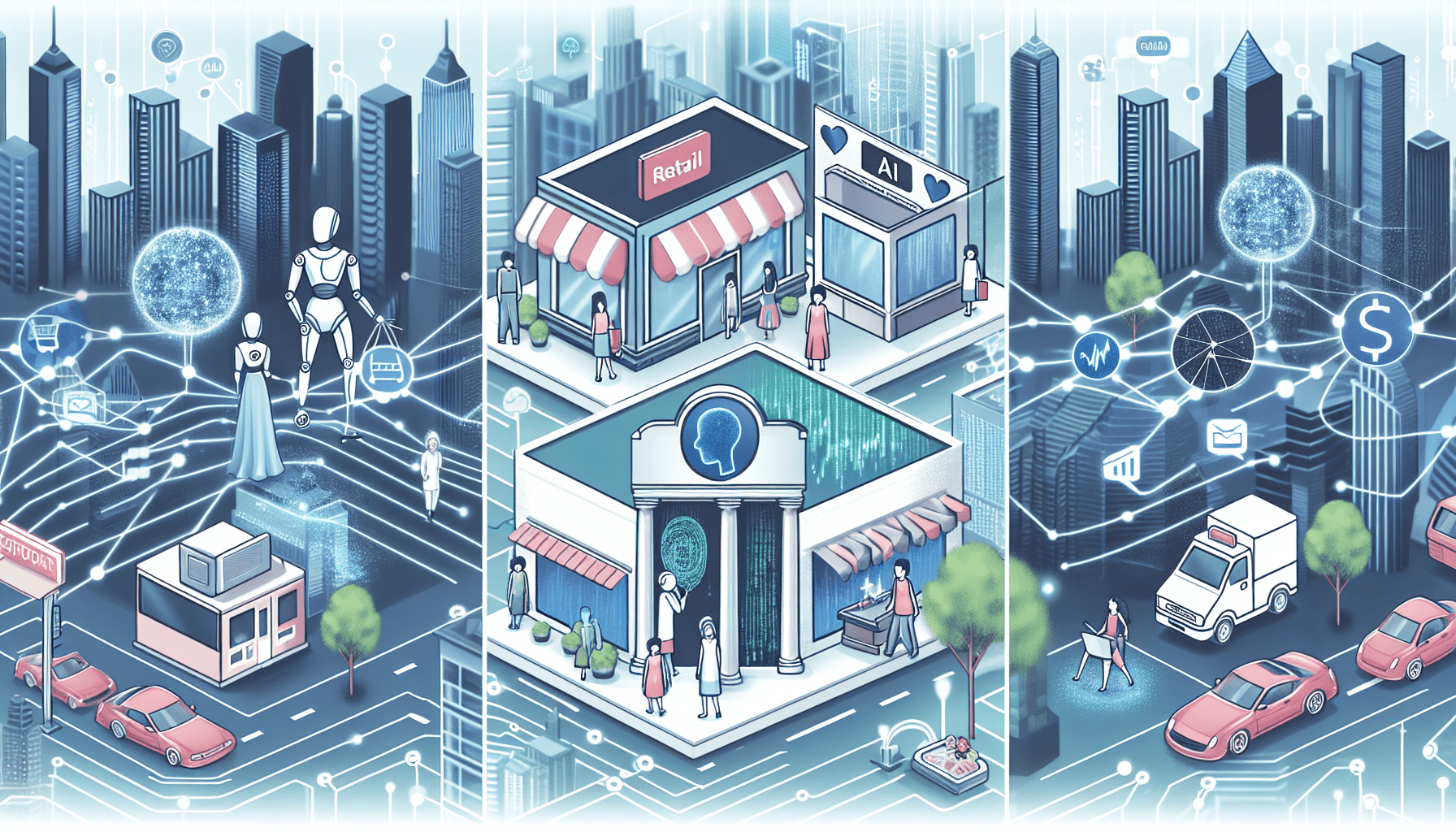
To truly understand the transformative potential of Generative AI and Large Language Models, let’s cover some real-world examples. From enhancing customer support in retail businesses to improving patient outcomes in healthcare and detecting fraud in the finance sector, these technologies are reshaping the landscape of various industries.
Retail and E-commerce
In the retail and e-commerce sector, AI has been a game-changer. AI has transformed the way businesses interact with their customers by:
-
Enhancing the customer experience: AI-powered chatbots, recommendation engines, and personalized marketing strategies have made shopping more efficient and enjoyable for customers. These AI-driven tools can understand customer preferences and behaviors, providing them with a personalized shopping experience that boosts satisfaction and loyalty.
-
Boosting customer satisfaction: AI can analyze customer feedback and reviews to identify areas of improvement. It can also predict customer behavior, enabling businesses to proactively address issues and exceed customer expectations, thereby boosting satisfaction.
-
Bolstering customer retention: By providing a seamless and personalized shopping experience, AI helps businesses build strong relationships with their customers. This not only increases customer satisfaction but also bolsters customer retention, which is crucial in today’s competitive retail landscape.
-
Enhancing customer service operations: AI-powered virtual assistants and chatbots can handle a wide range of customer service tasks, from answering frequently asked questions to handling returns and exchanges. This not only improves the efficiency of customer service operations but also ensures that customers receive immediate assistance, enhancing their overall experience.
Healthcare
In healthcare, AI has played a pivotal role in enhancing patient outcomes. AI has:
-
Identified health risks: By analyzing patient data and identifying patterns, AI can predict potential health risks and diseases, enabling early intervention and prevention.
-
Accessed medical data: AI can process and analyze vast amounts of medical data, from patient records to medical literature, providing healthcare professionals with valuable insights and aiding in diagnosis and treatment.
-
Personalized care: AI can tailor healthcare to the individual needs of each patient, from personalized treatment plans to targeted therapies, improving patient outcomes and satisfaction.
-
Improved the efficiency of healthcare delivery: AI can streamline healthcare operations, from managing patient appointments to automating administrative tasks, reducing wait times and improving patient experience.
-
Generated insights to improve patient care: AI can analyze patient feedback and outcomes to identify areas of improvement, enabling continuous improvement in patient care and healthcare services.
These advancements have significantly improved patient outcomes, making healthcare more predictive, personalized, efficient, and effective.
Finance
In the finance sector, AI has not only improved fraud detection but also enhanced informed financial decision-making. By processing vast amounts of data and identifying patterns, AI has enabled financial professionals to make more informed decisions, leading to improved operations.
Specifically, AI has transformed the way financial institutions handle fraud detection by using machine learning algorithms to identify unusual patterns or anomalies that may indicate fraudulent activity. This allows for real-time detection and prevention of fraud, significantly reducing financial losses and enhancing the security of financial transactions.
Moreover, AI has revolutionized financial decision-making by providing insightful data analysis. With AI, financial institutions can process and analyze vast amounts of data in real-time, identifying trends, patterns, and correlations that would be impossible for humans to detect. Financial professionals can make more accurate predictions, assess risks more effectively, and make strategic decisions based on data-driven insights.
In addition, AI has also helped to improve operational efficiency in the finance sector. Tasks that once required extensive manual labor, such as data entry and verification, can now be automated with AI, freeing up employees to focus on more strategic tasks and significantly reducing operational costs.
Overall, integrating AI in the finance sector has significantly improved fraud detection, decision making, and operational efficiency, making it a crucial tool for modern financial institutions.
Future Trends in AI
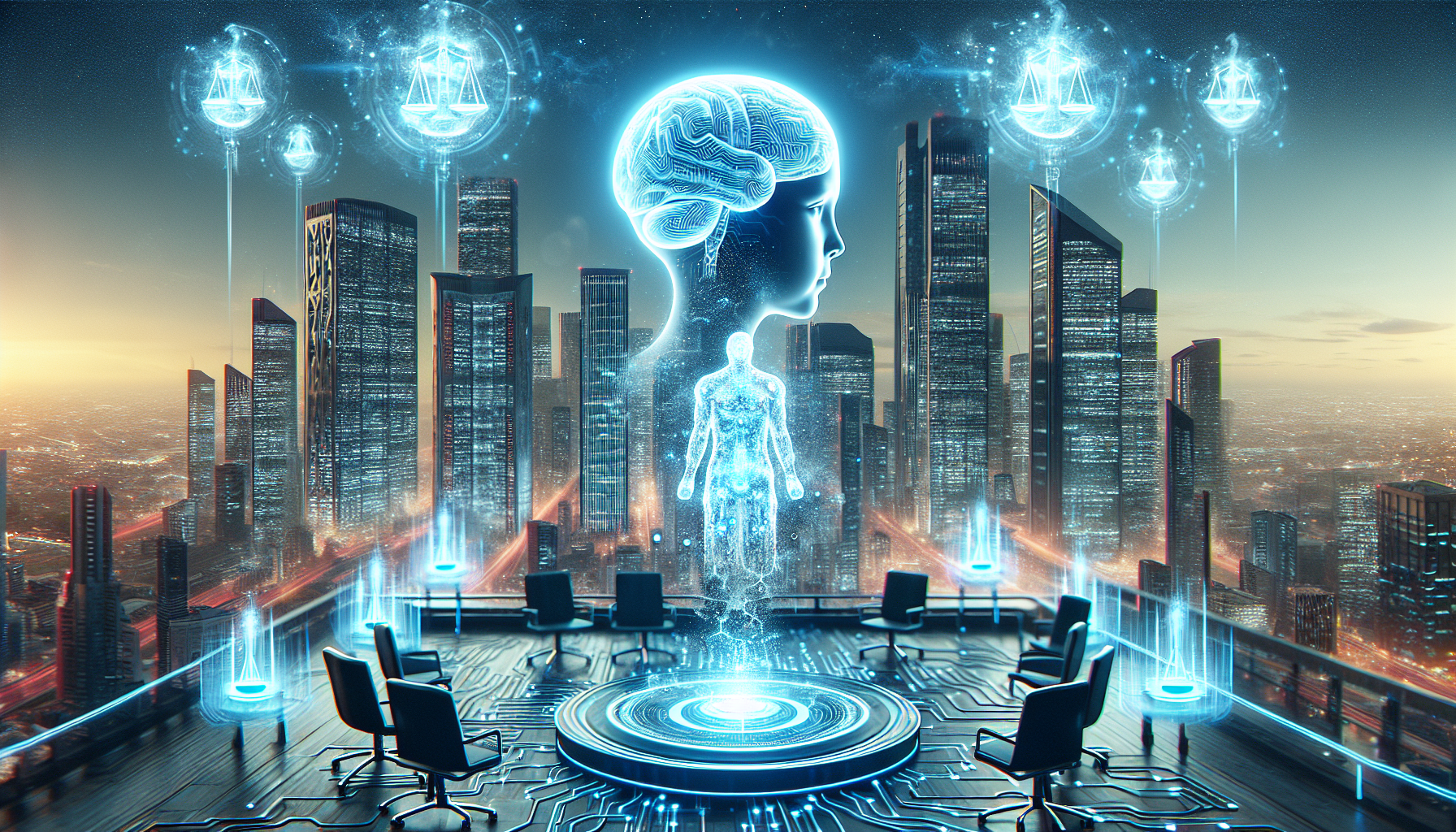
As we look to the future, AI continues to evolve, promising even more transformative capabilities. From AI-powered decision-making and integration with IoT and blockchain to a focus on ethics and responsible AI development, the future of AI holds immense potential in the realm of emerging technologies.
AI-Powered Decision-Making
One of the key trends in AI is the growing prevalence of AI-powered decision-making. AI analyzes extensive volumes of data and streamlines decision-making processes, enabling businesses to make more informed, data-driven decisions and thereby improve their operational efficiency through data driven decision making. This trend is revolutionizing industries across the board. For instance, in the healthcare sector, AI-powered decision-making tools can analyze patient data and medical research to aid in diagnosis and treatment plans. In the realm of finance, these tools can process and analyze market trends and historical data to aid in investment decisions. In the retail sector, AI can help businesses understand their customers better, predict buying patterns, and personalize their marketing strategies. Thus, AI-powered decision-making is not just a trend, but a powerful tool that is transforming the landscape of business operations, making them more efficient, effective, and informed.
Integration with IoT and Blockchain
The integration of AI with IoT and blockchain is another trend that is set to expand AI capabilities. This integration analyzes large datasets collected by IoT devices and provides transparency and security through blockchain, driving innovation and creating new opportunities for businesses.
In this convergence, AI plays a critical role in analyzing and interpreting the vast amount of data generated by IoT devices. This data, which can include everything from temperature readings to consumer behavior, can be processed in real-time, allowing for more accurate and timely decisions.
On the other hand, blockchain technology provides a secure and transparent platform for this data. Blockchain’s immutable and decentralized nature ensures that the data is trustworthy and free from manipulation, which is particularly important when it comes to sensitive information.
This synergy of AI, IoT, and blockchain allows for more efficient and secure processes, ranging from supply chain management to smart homes and cities. The integration of these technologies is opening up new avenues for innovation, enabling businesses to optimize their operations, create new business models, and provide enhanced services to their customers.
Focus on Ethics and Responsible AI
As artificial intelligence (AI) advances, it is important to focus on ethics and responsible AI development. This involves creating and implementing AI technologies with fairness, transparency, and accountability in mind. It is essential to ensure that AI systems are designed and used in an ethical and legal manner.
The growing prevalence of AI systems in our daily lives makes it even more crucial to prioritize ethics and responsible development. These systems can have a significant impact on our society – from decision-making algorithms to autonomous vehicles. It is, therefore, necessary to develop these systems based on a strong ethical framework. This includes avoiding the perpetuation of existing biases, respecting user privacy, and maintaining transparency in their processes and decisions.
Moreover, responsible AI development also entails considering the potential societal impacts of AI systems. This includes both positive and negative implications, such as increased efficiency and productivity, and job displacement due to automation, respectively. By taking these impacts into account during the development process, AI practitioners can create systems that maximize the benefits of AI while minimizing its potential harm.
Lastly, accountability in AI development is a key aspect of ethical AI. This means that AI practitioners must be accountable for the systems they create, which could involve auditing AI systems, establishing clear lines of responsibility for AI outcomes, and creating avenues for redress when AI systems cause harm.
Prioritizing ethics and responsible AI development is a critical aspect of AI’s evolution. By focusing on fairness, transparency, and accountability, we can ensure that AI systems are designed and used in a way that benefits society as a whole.
Summary
Generative AI and Large Language Models have immense potential to enhance efficiency, personalize customer experiences, transform industries and drive future trends. As businesses continue to adopt their use, it is crucial to prioritize ethical considerations, overcome challenges and ensure privacy and security to fully realize their benefits.
Integrating these technologies into various business operations is revolutionizing how businesses operate. They automate repetitive tasks, streamline workflows, and extract valuable insights from large datasets, freeing employees’ time to focus on more strategic and creative tasks. This increases productivity, employee satisfaction, and engagement.
Furthermore, these AI models’ personalization capabilities are taking customer experiences to a new level. By understanding and predicting customer behavior, businesses can offer more targeted and personalized services, increasing customer satisfaction and loyalty.
As with any powerful technology, some challenges need to be addressed. Data privacy and security are paramount, and businesses must adhere to all relevant regulations and best practices to protect their customers’ data. Ethical considerations are also crucial, and businesses must ensure that these AI models are being used responsibly and fairly.
The potential benefits of Generative AI and Large Language Models far outweigh these challenges. By overcoming these obstacles, businesses can unlock the full potential of these technologies and transform their operations for the better.
Interesting in Learning More?
Are you unsure of where to start when it comes to harnessing the potential of generative AI and Large Language Models? Look no further than InTech Ideas. Our team of experts specializes in assembling software development teams that can seamlessly integrate AI into their processes and applications. Don’t hesitate to reach out to us today for guidance.